BIG DATA CONFERENCE
EUROPE 2021
Online Edition
September 28-30
Online
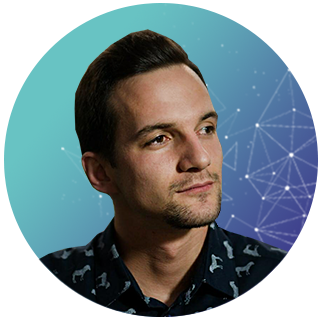
Ibrahim Muzaferija
Data Scientist
Maestral Solutions, Bosnia & Herzegovina
Biography
Creates links between academia and industry. Solves cross-industry problems with algorithms. Tells stories from data. Experienced as a researcher, software engineer, machine learning specialist, data scientist.
Talk
Cloud Computing Anomaly and Threat Detection Using Big Data Analytics and Machine Learning
While leveraging cloud computing for large-scale distributed applications allows seamless scaling, many companies struggle to follow up with the amount of data generated in terms of efficient processing and anomaly detection. With the rapid growth of web attacks, anomaly detection becomes a necessary part of the management of modern large-scale distributed web applications. As the record of user behaviour, weblogs certainly become the research object related to anomaly detection. Many anomaly detection methods based on automated log analysis have been proposed. However, not in the context of big data applications where normal and anomalous behaviour models need to be constructed before prediction attempts.
To address this problem, Big Data Analytics and Machine Learning algorithms in overcoming the challenges of data processing, pattern detection, and anomaly prediction in large and high-dimensional data representing user and application logs are utilized. Integrating CRISP-DM methodology, we propose PCA for dimensionality reduction and a combination of unsupervised machine learning algorithms: Random Isolation Forest and Global Homogenous Outlier Search for pattern detection and construction of labelled dataset, that is initial model of normal and anomalous behaviour. Next, a supervised machine learning algorithm one-class SVM is trained to generalize the behaviour model in order to predict user behaviour anomalies.
Results show that One-Class SVM is the most efficient supervised algorithm in generalizing the behaviour patterns and, as the algorithm is capable of anomaly detection, it improves the patterns detected by unsupervised models with a prediction accuracy of 99% and outlier class recall of 80%. We conclude that the use of unsupervised learning as a baseline improves the model ageing which occurs in real-world applications, and the one-class approach in supervised learning contributes to better pattern recognition.
Session Keywords
« Back