BIG DATA CONFERENCE
EUROPE 2021
Online Edition
September 28-30
Online
Abhishek Malik
Data Scientist
Germany, Hawk:AI
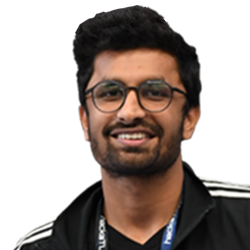
Biography
Abhishek is all about Data! He is passionate about Data Science, AI, and new technologies. He works as a Data Scientist at Hawk:AI, where he is building interactive and explainable AI systems to detect Fraud and Money Laundering in banking data. He enjoys talking about his academic background i.e. Physics and Astronomy. Recently, he also worked on ‘Planet Detection using Machine Learning’ where he built a novel machine learning based technique to detect planets which outperforms conventional algorithmic methods.
He is a self-taught programmer and enjoys building applications as side projects. In his most recent side project, he made a Python based tool that automatically inserts new content into Goethe Institute’s website by editing its HTML code in a systematic manner. This ensures that the content insertion process, which was done manually in about 50 hours, can now be done in a matter of seconds. Also, he recently got interested in DeepFakes and is currently developing an application in that area.
Apart from that, he also enjoys dancing Salsa, playing Tennis and drinking beer.
Talk
Exoplanet Detection Using Machine Learning
Speaker will introduce a new machine learning based technique to detect exoplanets using the transit method. Machine learning and deep learning techniques have proven to be broadly applicable in various scientific research areas. He aims to exploit some of these methods to improve the conventional algorithm based approach used in astrophysics today to detect exoplanets.
Abishek used popular time-series analysis library ‘TSFresh’ to extract features from lightcurves. For each lightcurve, we extracted 789 features. These features capture information about the characteristics of a lightcurve. He used these features later to train a tree-based classifier using a popular machine learning tool ‘lightgbm’.
This was tested on simulated data which proved it to be more effective than conventional box least squares fitting (BLS). It produced comparable results to the existing state-of-art models while being much more computationally efficient and without needing more processed versions of lightcurves such as folded and secondary views. On Kepler data, the method is able to predict a planet with an AUC of 0.948 which means that, 94.8\% of the time a planet signal is ranked higher than a non-planet signal and recall of 0.96 meaning, 96\% of real planets are classified as planets. With Nasa’s Transiting Exoplanet Survey Satellite (TESS), a reliable classification system is much needed as we are receiving over a million lightcurves per month. However, classification of this data is harder as lightcurves are shorter. His method is able to classify lightcurves with an accuracy of 98\% and its able to identify planets with a recall of 0.82.
Session Keywords
« Back