BIG DATA CONFERENCE
EUROPE 2022
November 23-24
Online
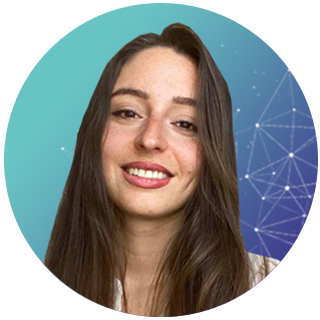
Diana Gabrielyan
Data Analytics Team Lead
Stockmann, Finland
Biography
Part-time data scientist, part-time data analyst, full time PhD candidate currently leading the data analytics team at one of the biggest retailers of Nordic-Baltics. Day-to-day work includes business intelligence, data science and econometrics. Past hands-on working experiences in various industries, including banking, government, airline and start-up.
Talk
Machine Learning Helping the Economy
Machine learning is a catalyst for productivity growth. In the near future, many current jobs and tasks will be performed totally by machine learning and Artificial Intelligence algorithms or with the usage of them. According to PWC, machine learning in economics can increase productivity by up to 14.3% by 2030.
We have heard and read about this a lot. What we do not hear a lot about is how machine learning applications help policymakers, economists and central bankers. We don’t talk enough about this possibility because we don’t have good practical examples. Applying machine learning requires strong mathematics and statistical skills, whereas traditionally economists did not need to have these skills. Closest to data scientists are econometricians, but it still does not mean that they use ML and more novel methods for the assessment and prediction of economic variables.
This is however changing. Academics are now actively researching how ML can be applied in macro and microeconomics. She is one of these academics.
Before she tells you about what she is doing, she wants to talk about a cool project that inspired her to do what she is doing now as part of her research. Two researchers from MIT – Alberto Cavallo and Roberto Rigobon have in 2008 founded the Billion Prices Project, which is an academic initiative that uses prices collected from hundreds of online retailers around the world on a daily basis to conduct research in macro and international economics. Noteworthy is that they started the project in 2008 when the term big data was not a buzzword.
One of the coolest applications and outcomes from this project, in her opinion, is to successfully estimate the actual inflation in Argentina. The story with Argentinian inflation is as follows: by 2007, it had become apparent that the official level of inflation reported by the national statistical office in Argentina did not reflect the actual changes in prices. Using online data collected every day from the websites of large retailers, Cavallo showed that while Argentina’s government announced an average annual inflation rate of 8 per cent from 2007–2011, the online data suggested it was actually over 20 per cent, in line with the estimates of some provincial governments and local economists, and consistent with the results from surveys of household inflation expectations. The online price indexes used in that paper were automatically computed and published on a website every day from March 2008 onwards. 2 The ability to collect prices from outside the country proved particularly useful in 2011 when Argentina’s government started to impose fines and to pressure local economists to stop collecting data independently. The manipulation of the official price index ended in December 2015 when a new government was elected. Currently, they are collecting data to accurately measure the inflation in Venezuela – a country that is in a very bad economic situation currently.
She personally thinks this is one of the best applications this technological advances can give us. Of course, having robots and self-driving cars is super cool, but unless we are able to accurately assess the economy and make predictions, technological progress and economic growth won’t be possible. Covid 19 showed us the importance of just that – it showed how important it is for policymakers to do timely and real-time analysis of the situation for the economy to react to any crisis faster and better. Had this been possible in 2008, who knows what the impact of the financial crisis would have been. She is guessing, less.
In her own research, she is using another branch of ML – text mining, to build a high frequency and real-time inflation measure, that captures true consumer expectations. The idea is that this metric presents the consumer’s perceptions about inflation more accurately than actual statistics collected through survey measures. The problem with the survey data is that the information is vast and costly to obtain. Consumers receive only very partial information while doing everyday shopping and build their expectations through personal experiences and prior memories, which however can be inaccurate, irrational and diverse.
To solve this problem, she turns to media outlets and using the textual data from news articles that are discussing the economy, she builds a quantitative measure of inflation that is quite correlated with actual inflation, except that it is a real-time measure and can therefore help central bankers to predict price changes faster.
Session Keywords
« Back