BIG DATA CONFERENCE
EUROPE 2021
Online Edition
September 28-30
Online
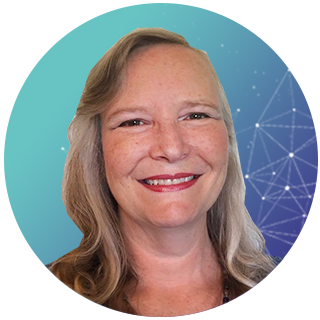
Paige Roberts
Open Source Relations Manager
Vertica, US
Biography
Paige Roberts has worked as an engineer, trainer, support technician, technical writer, marketer, product manager, and a consultant in the last 24 years. She has built data engineering pipelines and architectures, documented and tested open source analytics implementations, spun up Hadoop clusters, picked the brains of stars in data analytics, worked with different industries, and questioned a lot of assumptions. She’s worked for companies like Data Junction, Pervasive, Bloor Group, Hortonworks, Syncsort, and Vertica. Now, she promotes understanding of Vertica, distributed data processing, open source, high scale data engineering, and how the analytics revolution is changing the world.
Talk
In-Database Machine Learning with Jupyter
The data warehouse has been an analytics workhorse for decades. Unprecedented volumes of data, new types of data, and the need for advanced analyses like machine learning brought on the age of the data lake. But Hadoop by itself doesn’t really live up to the hype. Now, many companies have a data lake, a data warehouse, or a mishmash of both, possibly combined with a mandate to go to the cloud. The end result can be a sprawling mess, a lot of duplicated effort, a lot of missed opportunities, a lot of projects that never made it into production, and a lot of financial investment without return. Technical and spiritual unification of the two opposed camps can make a powerful impact on the effectiveness of analytics for the business overall.
Over time, different organizations with massive workloads like IoT have found practical ways to bridge the artificial gap between these two data management strategies. Look under the hood at how companies have gotten high scale machine learning projects working, and how their data architectures have changed over time. Learn about new architectures that successfully supply the needs of both business analysts and data scientists. Get a peek at the future.
In this area, no one likes surprises.
– Look at successful data architectures from companies like Philips, Anritsu, Uber, …
– Learn to eliminate duplication of effort between data science and BI data engineering teams
– Avoid some of the traps that have caused so many big data analytics implementations to fail
– See a variety of ways companies are getting AI and ML projects into production where they have a real impact, without bogging down essential BI
– Study analytics architectures that work, why and how they work, and where they’re going from here
Session Keywords
« Back